29/01/2021
With Artificial Intelligence becoming increasingly relevant to our daily lives, many inventors are looking to gain patent protection for their technology. As discussed in our previous insight here, there are extra considerations to bear in mind when seeking patent protection for an AI invention in Europe. However AI patent applications can be, and are being, granted at the European Patent Office.
Historical Grant Rates
AI related patent applications have been filed as far back as the 1980s, with numbers exploding in recent years. To investigate the success rate of AI related applications we looked at the Cooperative Patent Classification (CPC) codes assigned to past AI related applications. All European and UK patent applications are classified by subject using CPC codes, and it is usual for a patent application to be assigned to two or sometimes three CPC codes. The majority of AI related applications were classified as at least one of:
G06N 3/xx – Computer systems based on biological models
G06N 5/xx – Knowledge-based models
G06N 7/xx – Specific mathematical models
G06N 20/xx – Machine Learning
At the time of writing there were 11,581 European applications assigned to at least one of these four CPC codes. Out of these applications 2,040 (18%) have been granted by the EPO, 2,936 (25%) had ceased before reaching grant, and 6,605 (57%) remained pending.
It would be misleading to view the success rate of AI related patents as 18% though, as many of these applications have only been filed in the past year or two. A European patent application usually takes three to five years to progress from filing to grant (or refusal), and therefore many of the 6,605 pending applications will be granted in coming years.
This lag between filing and grant is perhaps best demonstrated by the graph below, which shows the number of AI related European patent applications filed and the number of patent applications granted through time. Whilst the rate of filing shows a dramatic increase since 2015, the grant rate is only just starting to show a similar increase. Based on the expected lag between filing and grant, and the recent uptake in grant rate (increasing from 156 in 2018, to 268 in 2019, and to 433 in 2020), it seems more than likely that the grant rate will continue to increase in the future.
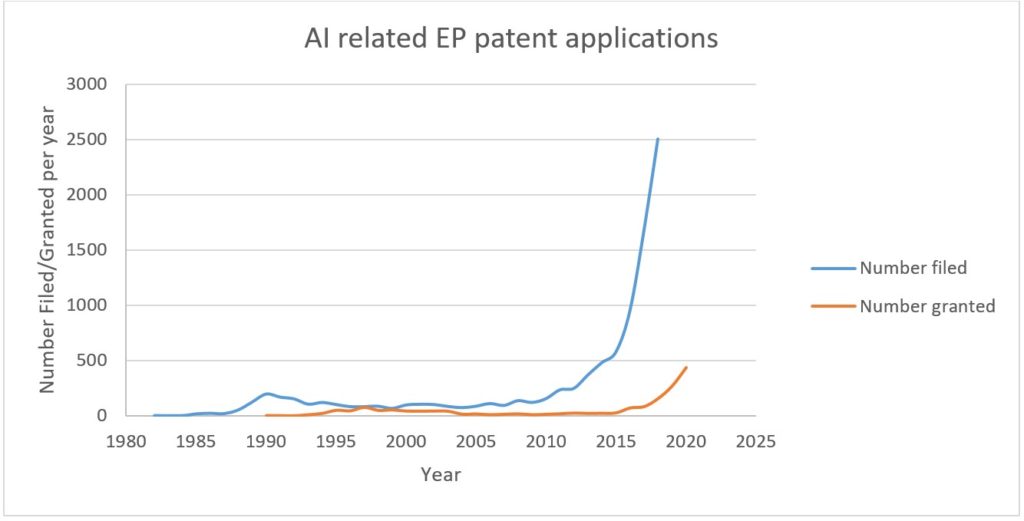
Figure 1: Number of European patent applications filed/granted per year in the four listed AI CPC classification codes.
The situation at the UKIPO appears to be broadly similar, with 1,041 AI related UK patent applications filed, of which 316 (30%) have been granted, 260 (25%) have ceased before being granted, and 465 (45%) remain pending.
Examples of Granted European Patents
The applicants in our data set having the most granted AI related EP patents include many of the big names you would expect, such as Google, Hitachi, Microsoft, Mitsubishi, Fujitsu, Qualcomm and Samsung, to name a few. Siemens had the most granted EP AI patents, with 112 in total. A small selection of granted Siemens patents are discussed below, to provide examples of the types of subject matter that can lead to a granted patent.
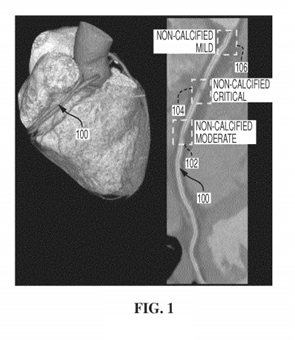
EP3261024B1 relates to use of neural networks in medical imaging, and figure 1 of the patent is reproduced above. Claim 1 can be approximated as:
“a method for vascular disease detection using a recurrent neural network, comprising:
extracting a plurality of 2D cross-section image patches at a plurality of sampling points along a centreline of a vessel of interest in a 3D computed tomography angiography image;
detecting vascular abnormalities in the vessel of interest by encoding each of the 2D cross-section image patches into a feature vector using a trained convolutional neural network, CNN; and classifying each of the plurality of sampling points based on the feature vectors using a trained bi-directional recurrent neural network, RNN.”
In this case, the application of the neural networks to the field of medical image analysis helped ensure that the claims passed the European Patent Office’s technical character test and were not deemed simply abstract algorithms which would not be patentable. The combination of both a convolutional neural network and a trained bi-directional recurrent neural network was sufficient to persuade the examiner of an inventive step.
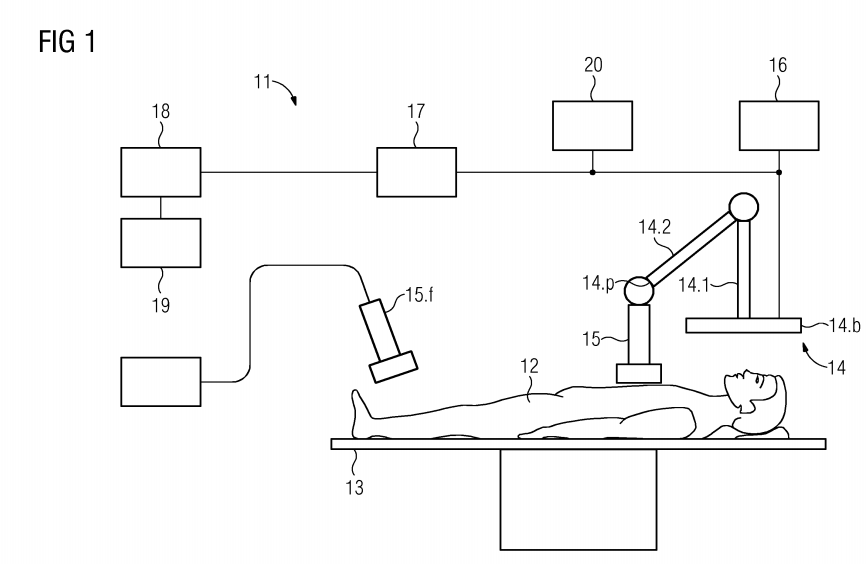
EP3409230B1 demonstrates some of the problems of classification based searches. It relates to a method for moving a robotic arm during an ultrasound procedure and uses a trained artificial neural network to determine a motion dataset for directing motion of a robot arm to conduct an ultrasound examination. You might expect that training the artificial neural network or using it to determine the motion dataset would be a key feature. In fact, the application includes very little in the way of training details or detail of the artificial neural network. The feature which was judged novel and inventive was that the motion sequence (for the robot arm) is projected onto the surface of the patient’s body by means of a projector. Whilst the patent demonstrates the type of application that AI is being used for, it is not an example of a patent which hinges on AI.
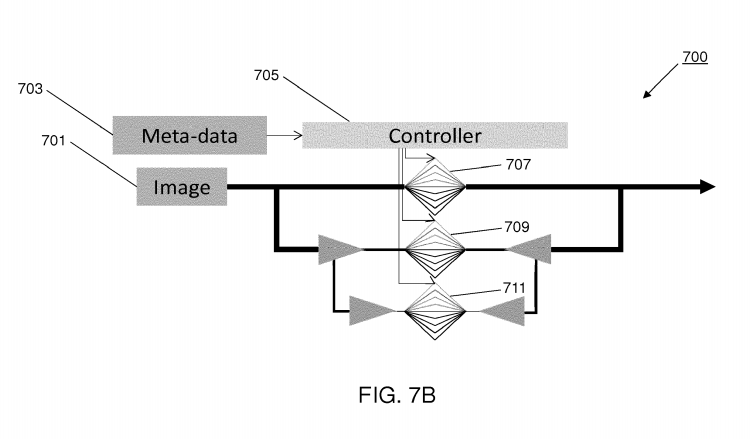
EP3367329B1 is more interesting from an AI perspective. It relates to a method of removing noise from a medical image and, amongst other features, requires:
“denoising computer tomography (CT) image data by applying a deep-learnt multiscale network of filters decomposing the CT image data into sparse image representations at multiple scales, the deep-learnt multiscale network of filters comprising a cascade of trained sparse denoising autoencoders, wherein lower levels of the deep-learnt multiscale network apply learnt filters to image data recursively downsampled from the CT image data and resulting denoised image data at each scale is upsampled back to full resolution and summed to obtain a final denoised image CT data set; and the inputs to the sparse denoising autoencoders are a concatenation of CT pixel data and metadata of the CT system.”
Claims relating to image processing are typically seen as being technical in character at the EPO, and the granted claim included scanning and displaying steps to link the AI features to a particular technical field. The CT pixel data and metadata (such as scan settings including dose or x-ray source voltage) are provided as an input to the autoencoders during their training and are at the heart of the deep learning which is credited with allowing the network to adapt to different scan parameters. In this case, the AI related aspect of the application is core to the granted patent.
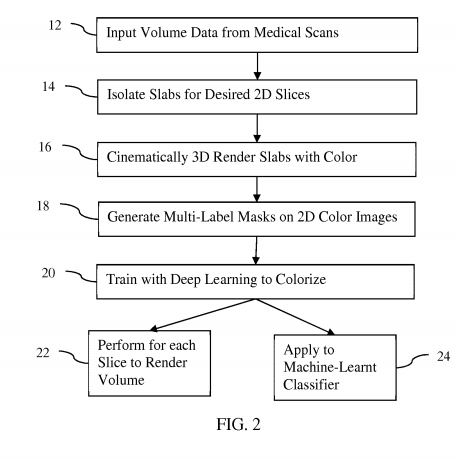
As well as methods using AI to solve particular problems, a method of training an AI may also be protected. Also in the field of medical imaging, the broadest claim of EP3296962B1 relates to training a deep machine-learnt classifier and requires:
“A method for training (20) a deep machine-learnt classifier for colorizing a two-dimensional medical image, the method comprising:
loading (12) to a memory data representing volumes of multiple patients, the volumes comprising scalar values distributed in three dimensions from medical scanning of the patients;
isolating (14) slabs each representing multiple slices in each of the volumes;
three-dimensional color rendering (16) the scalar values of the slabs to two-dimensional color images with cinematic rendering (16);
training (20), from the two-dimensional color images, a deep machine-learnt classifier with deep machine learning and colorizing the two-dimensional medical image.”
This training related application was granted with minor amendments only six months after examination commenced (albeit three years after filing).
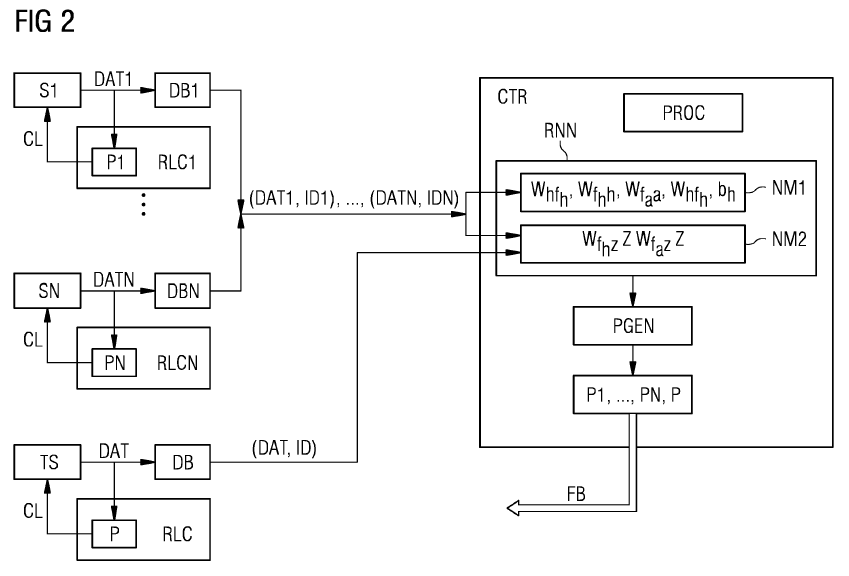
A last example shows that an AI based control method applied to a non-specific system can be patentable where an improvement to the functioning of a computer is achieved. EP3117274B1 relates to a way of controlling a system which allows more rapid learning of control strategies. Gas or wind turbines are given as examples where the invention may be used but the patent protection is not application specific. Rather, it concerns the interplay of pre-training a neural network with operational data from several similar systems with training specific to operational data from the system to be controlled. Claim 1 of EP3117274B1 can be simplified and paraphrased as:
A method for controlling a target system based operational data of a number of source systems, comprising:
a) receiving operational data of the source systems
b) training by means of a neural network a neural model on the basis of the received operational data, where a first neural model component is trained on properties shared by the source systems and a second neural model component is trained on properties varying between the source systems,
c) receiving operational data of the target system,
d) further training the trained neural model on the basis of the operational data of the target system, where a further training of the second neural model component is given preference over a further training of the first neural model component, and
e) controlling the target system by means of the further trained neural network.
The European Patent Office was persuaded that the application should be granted because features b) and d) provided the technical effect of avoiding unnecessary training on well-fitted models, therefore reducing the computation load during training of the neural network.
Summary
As demonstrated by the examples above, European patent protection can be obtained for various aspects of AI related technology. Moreover, we expect to see more AI patent applications getting granted in the future as this technology continues to develop.
At Reddie and Grose we have a wealth of experience in artificial intelligence and computer implemented technologies. If you would like help protecting your innovations in these fields then please get in touch.
This article is for general information only. Its content is not a statement of the law on any subject and does not constitute advice. Please contact Reddie & Grose LLP for advice before taking any action in reliance on it.